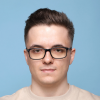
Online Shopping Recommendations – Introducing Them to Your Business
Wondering what good product recommendations should look like on an online storefront?
This article explores the best practices for integrating personalized recommendations into your ecommerce platform to improve the user experience and drive sales.
By understanding how these recommendation engines work and the value they provide, you can effectively implement them in various areas of your site, from product pages to the shopping cart.
What are Product Recommendations?
Product recommendations engines are a critical feature of modern e-commerce platforms, leveraging sophisticated algorithms and vast amounts of data to personalize the shopping experience for users.
These recommendations are designed to suggest products that match a customer’s preferences, browsing history, and purchase behavior, thereby increasing user engagement, driving sales, and increasing average order value.
Personalized recommendations work by analyzing individual user data and comparing it with the behavior of similar users on the ecommerce site, often employing machine learning techniques to refine and optimize suggestions.
This approach not only improves satisfaction of online shoppers by making it easier to discover relevant products but also significantly increases conversion rates for businesses.
The popularity of personalized recommendations is evident in their widespread adoption by major e-commerce and media giants like Amazon, Netflix, and Alibaba, demonstrating their effectiveness in driving user interaction and sales growth.
How Product Recommendations Should Look Like in the Ecommerce Store?
Effectively integrating product recommendations into an e-commerce store’s user interface requires a delicate balance between personalization and user experience (UX).
The goal is to enhance the shopping journey without overwhelming or distracting the customer. Well-executed personalized recommendations should feel like a natural extension of the browsing experience, adding value and relevance at every touchpoint.
Key considerations for integrating these elements into the store UI include relevance, context, and unobtrusiveness, ensuring that recommendations enhance rather than detract from the UX.
Recommendations should be contextually appropriate, appearing where they make sense and add real value. For example, displaying complementary items on a product page or suggesting related products after an item has been added to a shopping cart can help users find what they need without additional effort.
Personalized recommendations also need to be seamlessly integrated into the user interface, while maintaining a clean and intuitive design. They should enhance the browsing experience without overwhelming the user with too many options or cluttering the interface.
Here are some of the best places to implement these recommendations:
Product page
The product page is by far the most powerful location for product recommendations.
Placing a recommendation banner below helps customers discover complementary or alternative products, enhancing their shopping experience and increasing the likelihood of additional purchases.
The best product recommendation examples are: Similar Products, Other Customers Also Purchased, Frequently Bought Together, Recently Visited In Store.
Category page
Within category pages, you can display personalized product recommendations or popular items based on a shopper’s browsing history or preferences, guiding them to products they may have overlooked.
Recommendations like “Top picks for you” or “Trending in this category” can help streamline their search and reduce decision fatigue.
Pop-up after adding a product to the shopping basket
A well-timed pop-up after adding an item to the cart can suggest related products or accessories. For example, if a customer adds a laptop to their cart, a pop-up could recommend a laptop bag or mouse.
This encourages impulse buys and increases the average order value without disrupting the shopping experience.
To show your consumers relevant products, consider these product recommendation examples: You May Also Like, Other Customers Also Purchased, Bestsellers, Frequently Bought Together.
Store Home Page
The home page is ideal for relevant recommendations based on previous interactions, as well as displaying some of your best selling products.
Featuring sections like “Recommended for you,” “Recently viewed,” or “New arrivals you might like” can create a tailored shopping experience from the moment the customer lands on the site, enticing them to explore further.
Pop-up on the page of an unavailable product
The recommendation system helps to redirect the traffic from an unavailable product to other pages of the store with available products.
The pop-up displays similar items to the unavailable one and the most popular items in the store (models: Bestsellers in Category, Similar Products).
Cart page
On the cart page, suggestions such as “You might also like” or “Complete the look” can encourage last-minute additions before checkout.
These recommendations should be relevant to the items in the cart, providing a final opportunity to increase the basket size while ensuring the customer feels their needs are understood increasing the long term customer loyalty.
What Value Does Personalization Provide to Ecommerce Companies?
Personalization in e-commerce is a powerful tool that can transform the shopping experience and deliver significant benefits to companies that implement it effectively.
By tailoring the online journey to individual preferences and behaviors, e-commerce companies can achieve greater customer satisfaction, loyalty and, ultimately, improved business performance.
Here are six key benefits of integrating personalized recommendation engines into e-commerce sites:
Enhanced Customer Experience
Personalized recommendations create a more engaging and relevant shopping experience.
By displaying products that match a customer’s tastes and needs, businesses can make the shopping process smoother and more enjoyable, resulting in higher customer satisfaction and repeat visits.
Increased Conversion Rates
Tailored product suggestions can significantly increase conversion rates. When customers see items that match their preferences, they are more likely to make a purchase. Personalization helps bridge the gap between browsing and buying, resulting in more sales.
Higher Average Order Value
Personalized recommendations often encourage customers to add complementary or additional items to their shopping carts. This up-selling and cross-selling strategy can increase the average order value, maximizing the revenue generated from each transaction.
Improved Customer Retention
By consistently offering relevant and valuable recommendations, e-commerce companies can build stronger relationships with their customers.
This leads to increased customer loyalty and a higher lifetime value, as satisfied customers are more likely to return and make repeat purchases.
Efficient Inventory Management
Personalization engines can help companies better understand customer preferences and trends, enabling more accurate demand forecasting.
This can lead to more efficient inventory management, reducing overstock and stockouts, and optimizing the supply chain.
Actionable Insights and Analytics
Implementing personalization engines provides valuable data and insights into customer behavior and preferences. E-commerce companies can use this information to refine their marketing strategies, improve product offerings, and make informed business decisions, driving overall growth and efficiency.
By leveraging the power of personalization, e-commerce companies can create a more compelling and effective shopping experience, driving business success through increased sales, customer loyalty, and operational efficiency.
Want to reap the same benefits in your own e-commerce business?
Empower Your Business With AI-Driven Recommendation Engine Today!
Unlock the Power of Personalization with Custom Recommenation Engine
So you want to unlock the power of personalization in your ecommerce business, but you don’t know where to start?
There are countless off-the-shelf tools available to integrate with your business in 2024, some more robust than others.
But there’s one problem: they don’t address the complex needs of your business.
Out-of-the-box recommendation solutions often fall short due to their one-size-fits-all approach, which may not align with the unique needs and data of individual organizations.
These solutions may lack the flexibility to seamlessly integrate with existing systems, resulting in suboptimal performance and user experience.
In addition, they may offer limited customization options, preventing organizations from fine-tuning algorithms to address specific customer behaviors and business goals.
To address these issues, you may want to consider custom recommendation engines – software solutions that are perfectly tailored to your business scenario to deliver the most value and business impact.
To craft such a system work only with the best custom software development companies like Stratoflow that will understand your needs.
These companies specialize in creating tailored solutions that meet the unique needs of their clients.
At Stratoflow, we have extensive experience in developing custom personalization engines, exemplified by our flagship product, Recostream.
Our goal was to increase user engagement and drive sales through highly accurate, personalized recommendations.
And that’s how Recostream was born – a state-of-the-art AI/ML-driven ecommerce product recommendation engine aimed at improving online shopping experience.
Our solution not only increased sales by 5-10% for most clients, but also provided deep integration with Google Analytics, enabling robust performance tracking and A/B testing.
The system delivers recommendations within 20-30 milliseconds, ensuring high efficiency and customer satisfaction.
We specialize in building personalized recommendation engines not only for e-commerce, but for a wide range of industries.
Our expertise spans multiple industries, providing customized solutions that increase user engagement and drive business success.
Here are just a few of the industries we serve:
- Media and Entertainment: Enhance user engagement and retention with tailored content recommendations, ensuring users find relevant movies, shows, music, or articles that match their tastes.
- Education: Offer customized learning pathways that adapt to each student’s pace and learning style, improving educational outcomes and learner satisfaction.
- Healthcare: Provide personalized treatment plans based on patient data, improving healthcare outcomes and patient satisfaction through tailored medical advice and interventions.
- Insurance: Offer personalized financial advice and risk assessments, improving customer service and satisfaction by addressing individual needs and preferences.
- Finance: Provide tailored investment and financial advice, helping clients make informed decisions that align with their financial goals and risk tolerance.
- Food Delivery: Present personalized menu options and dynamic pricing, enhancing user experience and increasing order frequency by catering to individual tastes and preferences.
- Real Estate: Recommend properties and market analyses based on user preferences, improving the home-buying experience and helping clients find their ideal property.
At Stratoflow, our process involves working closely with clients to understand their specific needs and goals.
We start with an initial consultation to understand the project requirements and scope. We then conduct a detailed analysis to develop a strategy and select appropriate technologies. Next, we prepare a proposal outlining the scope of work, timeline, and cost.
During the development phase, we work in short development cycles and maintain constant contact with project stakeholders to ensure alignment and rapid iteration.
Ready to enhance your business with a custom personalization engine?
Contact us today to discuss your needs and how we can help you push personalization to the next level.
Personalizing the Appearance of Recommendation Banners
Customizing the look and presentation of recommendations on a online store page is crucial for enhancing user experience and driving engagement.
Personalized recommendations need to be not only accurate, but also visually appealing and seamlessly integrated into the overall site design. By customizing the display, businesses can ensure that recommendations are in line with their brand aesthetic, making them more appealing and more likely to be clicked on by users.
At Stratoflow, our developers excel at creating custom recommendation displays that fit perfectly with your site’s design language and enhance the online shopping experience.
We can customize the layout, style, and placement of recommendation widgets to match your brand’s look and feel. Whether it’s using specific colors, fonts, or animation effects, our team ensures that the recommendations enhance the user interface without disrupting the shopping experience.
In addition, we can implement a variety of presentation strategies, such as carousel sliders, grid layouts, or pop-up relevant recommendations, depending on what works best for your platform.
What’s Under the Hood: How Do Recommendation Engines Work?
Now that we’ve explored the what and how of personalized product recommendations, let’s take a closer look at the inner workings of these systems, drawing on our extensive experience with Recostream.
Understanding how these engines work can help e-commerce business owners implement them effectively to improve the user experience and drive sales.
We’ll explore the main models used by recommendation engines: collaborative filtering, content-based filtering, and hybrid models.
Collaborative Filtering Method
Collaborative filtering is one of the most common methods used to generate personalized product recommendations.
It works on the principle that users who have agreed on items in the past will agree on items in the future. This method can be further divided into two main types: user-based and item-based collaborative filtering.
- User-based Collaborative Filtering: This approach finds users who have similar preferences to the target user and recommends items that these similar users have liked. The system calculates the similarity between users using techniques such as Pearson correlation or cosine similarity, creating a user-item interaction matrix based on their purchase history. Based on this matrix, the system predicts which items the target user might like by looking at the preferences of similar users.
- Item-based Collaborative Filtering: Instead of focusing on user similarity, this approach looks at the similarity between items. It identifies items that are often rated similarly by users and recommends items that are similar to those the target user has interacted with. This method tends to be more scalable and stable over time since item similarities do not change as rapidly as user preferences.
Value of Collaborative Filtering
Collaborative filtering can uncover complex patterns and relationships in user behavior, making it highly effective for generating relevant recommendations.
However, it requires a large amount of user interaction data and can struggle with the “cold start” problem, where new users or items lack sufficient data to generate accurate recommendations.
Content-Based Filtering
Content-based filtering uses the attributes of items to make recommendations.
It analyzes the characteristics of items a user has interacted with and recommends similar items based on those characteristics.
It uses techniques from information retrieval and natural language processing to process and understand the content of items.
- Feature Extraction: The first step involves extracting relevant features from items. For instance, in a movie recommendation system, features might include genre, director, actors, and plot keywords.
- User Profile Construction: The system then constructs a user profile based on the features of items the user has previously liked or interacted with. This profile represents the user’s preferences in terms of item attributes.
- Similarity Calculation: The system calculates the similarity between the user’s profile and potential items using similarity measures such as cosine similarity or Euclidean distance. Items with high similarity scores are recommended to the user.
Value of Content-Based Filtering
Content-based filtering can effectively recommend items to users with unique tastes or interests since it focuses on the specific attributes of items.
It also mitigates the cold start problem to some extent, as it only requires item features to generate recommendations. However, it may struggle to recommend diverse items if user profiles become too narrow or specific.
Hybrid Models
Hybrid models combine elements of both collaborative and content-based filtering to leverage the strengths of each approach and mitigate their weaknesses.
These models can integrate multiple recommendation techniques in various ways, such as:
- Weighted Hybrid: Combines the scores from both collaborative and content-based methods, assigning different weights to each score based on their accuracy or relevance.
- Switching Hybrid: Switches between collaborative and content-based methods depending on the context, such as using content-based filtering for new users and collaborative filtering for established users.
Value of Hybrid Models
Hybrid models provide a more comprehensive and robust recommendation system by combining the strengths of multiple methods.
They can provide more accurate and diverse recommendations, address the cold-start problem more effectively, and adapt to different user contexts and data availability.
Conclusion
Custom personalization engines are invaluable tools for e-commerce, enhancing the shopping experience with tailored product recommendations that drive engagement and sales.
These systems provide accurate and relevant suggestions based on individual user preferences.
Ultimately, effective implementation of recommendation engines can lead to increased customer satisfaction, higher conversion rates, and improved business performance.
Related Posts
- Amazon Product Recommendation System: How Does Amazon Algorithm Work?
- Movie Recommendation Systems: A Business Guide
- How to Build a Recommendation System: Explained Step by Step
- How to Build an Inventory Management System: Key Steps and Tips
- How to Build a Document Management System: Alternative Approach
Thank you for taking the time to read our blog post!