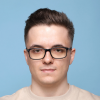
Amazon Product Recommendation System: How Does Amazon’s Algorithm Work?
The Amazon Product Recommendation System is a cornerstone of the eCommerce giant’s success, continually evolving to provide unparalleled personalization.
This sophisticated algorithm not only drives sales by suggesting relevant products but also enhances the overall shopping experience.
Discover how these cutting-edge techniques can be leveraged by businesses of all sizes to boost their own sales and customer satisfaction.
Contents
- What is The Amazon Recommendation System?
- How does Amazon use artificial intelligence in sales?
- How Amazon Leverages Data For Better Personalization
- How Does Amazon’s Recommendation Engine Work?
- Key Methods of Filtering Data By The Amazon’s Recommendation System
- Amazon sales results thanks to recommendations
What is The Amazon Recommendation System?
E-commerce recommendation systems are sophisticated AI/ML algorithms designed to enhance the shopping experience by predicting and suggesting products that customers are likely to purchase.
These systems analyze vast amounts of data, including past purchases, browsing history, and customer reviews, to generate personalized recommendations.
Amazon’s recommendation system is a prime example of this technology’s effectiveness in driving sales and customer satisfaction.
Leveraging machine learning and artificial intelligence, Amazon’s system continuously learns from user interactions, refining its suggestions to better match individual preferences.
This personalization not only boosts conversion rates but also fosters customer loyalty by creating a more engaging and tailored shopping experience.
Through collaborative filtering, content-based filtering, and hybrid models, Amazon’s recommendation engine successfully identifies patterns and trends, offering users a seamless and intuitive journey from discovery to purchase.
How does Amazon use artificial intelligence in sales?
How does the Amazon recommendation system work in practice?
Its concept is fairly simple on the surface.
Amazon’s AI-driven recommendation engine suggests products based on individual browsing history, past purchases, and items frequently bought together, significantly increasing the likelihood of sales.
Even though many of us don’t pay much attention to these personalized offers, subconsciously we rely on them more than we might assume.
According to the latest research on personalization, up to 91% of online store customers claim that they are more likely to use a brand’s offer that personalizes their experience. On the other hand, 98% of eCommerce website owners say that personalization improves their relationships with customers.
Whether it is improving your click-through rate, increasing the number of views, or reducing your bounce rate – personalization is an invaluable tool in working on improving these key metrics.
For this purpose, Amazon uses artificial intelligence in various areas of its business.
Amazon’s recommendation algorithm is therefore a key element in using AI to improve the personalization of the website.
[Read also: Inside the Netflix Algorithm: AI’s Role in Personalizing User Experience]
How Amazon Leverages Data For Better Personalization
Let’s take step back and take a closer look at how ecommerce recommendations, like the one Amazon uses, really work.
The online shopping behemoth uses its own custom system called A10 – a renowned version of its trusted A9 algorithm. It analyzes and classifies individual brands and their products on the platform, thanks to which it can offer Amazon customers relevant and personalized search results.
This system also serves as the basis for determining which sellers will be featured to buyers on the home page.
The Amazon A10 recommendation system operates based on three fundamental principles:
- Search Query Interpretation (NLP) – The A10 algorithm begins by interpreting user queries using advanced natural language processing (NLP) techniques. It goes beyond simple keyword matching, employing NLP to understand the semantic meaning and context of the search, ensuring that search results align with user intent. This involves analyzing the structure of the query and identifying relevant keywords, allowing the system to better match products that are most relevant to what the user is looking for.
- Relevance (Search and Ranking System) – Relevance is determined by analyzing product metadata such as titles, descriptions, and backend search terms. The algorithm prioritizes keyword placement and looks at how well a product’s text data aligns with the user’s query.
- Engagement and User Interaction Metrics – Engagement metrics, including click-through rate (CTR), conversion rate (CR), and session duration, are critical factors in ranking products. The A10 algorithm uses these metrics to assess how users interact with products and adjust rankings accordingly. Products that generate higher user engagement are given more visibility.
[Read also: Movie Recommendation System: How It Works And How To Introduce It In Your Business]
How Does Amazon’s Recommendation Engine Work?
To provide customers with precise product recommendations, Amazon’s A10 algorithm must process vast amounts of data.
By doing so, the algorithm gains a comprehensive understanding of overall user behavior and the specific interests of individual viewers.
This extensive data analysis enables Amazon to tailor its recommendations more effectively, ensuring that each customer is presented with products that align closely with their preferences and previous interactions on the platform just like media streaming platforms like Netflix and Spotify are tailoring content to their users.
Step 1: Gathering Data and Valuable Insights
The recommendation engine collects two main types of information:
- general data about products and users;
- data on relations and dependencies between them.
Getting to know the existing relationships in the online store will provide the recommendation engine with an insight into the real mechanisms governing customers’ purchasing decisions.
Amazon’s recommendation algorithm analyzes 3 main types of dependencies and relationships for its operation:
User-product
This type of relationship is observed when users with certain characteristics show a preference for specific types of products and purchase them more frequently.
An example of this would be gamers who frequently buy high-end computer components, or fans of various series and movies who purchase related gadgets and t-shirts.
Product-product
Product-product relationships exist when the items offered in the store share similarities in both appearance and specifications.
Examples include books, movies, series, or music within the same genre, or dishes from the same cuisine.
User-user
This relationship occurs when individual customers with specific characteristics have similar tastes or preferences for particular products.
For instance, teenagers might collectively purchase merchandise from their favorite YouTuber, or cooking enthusiasts might favor a particular line of kitchen products.
Additional Data
In addition to collecting information about relationships and connections, Amazon’s recommendation algorithm also utilizes various types of product and user data:
- User behavior data: This data includes valuable information about individual customers’ preferences and their interactions with products. Amazon collects this data using cookies to track:
- Browsing history
- Likes
- Session length
- User Demographics data: This data is connected to personal information about individual customers, such as:
- Age
- Education
- Income
- Location Collecting this data requires the user’s consent.
- Product Attribute Data: This data pertains to the product itself and includes details such as:
- Computer specifications
- Blouse size information
- Collection descriptions
Step 2: Filtering Data
Understanding the key methods of filtering data used by Amazon’s recommendation system provides valuable insights into how personalized shopping experiences are created.
These methods, including collaborative filtering, content-based filtering, and hybrid models, enable Amazon to deliver highly relevant product suggestions.
Let’s delve into how each method contributes to Amazon’s success in engaging customers and driving sales.
Content-based filtering
Content-based filtering is a fundamental method frequently employed by modern recommendation systems.
The core concept of content-based filtering is that if a customer enjoys a particular product, they are likely to appreciate another product with similar characteristics.
This method analyzes the attributes of products that a user has shown interest in and suggests items with comparable features. By focusing on product specifications and user preferences, content-based filtering provides tailored recommendations that align closely with individual tastes.
Collaborative filtering
Unlike content-based filtering, group filtering uses the experience of other users to generate recommendations.
Interestingly, Amazon pioneered this approach and published the article Recommendations: Item-to-Item Collaborative Filtering in 2003, which later won an award from the Institute of Electrical and Electronics Engineers (IEEE).
The undoubted advantage of this method is that it allows the recommendation engine to generate proposals for relatively complex products, such as movies or music, without the need to have specialist knowledge about them.
Compared to content-based filtering, team filtering produces better results in several key areas:
- diversity – group filtering generates a more diverse list of recommended products, offering customers a wider choice,
- randomness – recommendations generated using the collaborative filtering method are much more likely to positively surprise the client and show them a product of interest, which they might not otherwise discover,
- randomness – the collaborative filtering method is able to more effectively present to customers novelties in the store’s offer that users would most likely be interested in.
Hybrid Models
There are also hybrid models – combining the characteristics of the two previous approaches.
Amazon famously leverages two main types of hybrid recommendation models:
- Bandit-based algorithm: The so-called “bandits” algorithm utilizes reinforcement learning (RL) to enhance sales opportunities for new products by leveraging already successful ones. Additionally, Bandit-based algorithms can be employed to make real-time decisions between various product recommender models based on user responses to different product suggestions.
- Casual interference algorithm: This algorithm primarily focuses on identifying the factors that influence individual customers to notice specific products. By integrating casual interference with existing recommendation algorithms, Amazon researchers have developed improved recommendations that account for various confounding factors.
It is also noteworthy that hybrid systems are gaining popularity. Some of these new approaches are not mutually exclusive and can be combined.
All the strategies mentioned here are actively being developed and refined by the Amazon team to provide the best product recommendations to customers.
[Read also: 7 Best Books & 4 Research Papers on Machine Learning Recommender Systems]
Amazon sales results thanks to recommendations
Implementing such a complex and advanced recommendation algorithm required a substantial investment of both effort and funds from Amazon.
However, the statistics clearly show that this investment brings huge benefits.
Amazon’s remarkable success in the online marketplace demonstrates that the recommendation system is incredibly effective.
According to Statista during the first quarter 2024, Amazon generated total net sales of over 143 billion U.S. dollars, surpassing the 127 billion U.S. dollars in the same quarter of 2023.
Undoubtedly, a significant portion of this success can be attributed to Amazon’s seamless integration of shopping recommendations at nearly every stage of the purchasing process.
Source: Statista
What’s more, according to the McKinsey study, up to 35% of Amazon’s sales are generated thanks to the proprietary complementary product recommendation algorithm.
Currently, the recommendation engine has become a very important part of Amazon’s development strategy.
Jeff Wilke, director of the Consumer Division, admits:
At Amazon.com, we use recommendation algorithms to personalize the online store for each customer. The store changes radically based on customer interests, showing programming books to an engineer and baby toys to a new mom.
[Read also: How to Build a Recommendation System: Explained Step by Step]
Learn From Amazon’s Success: Advantages of Ecommerce Recommendation Systems
The most important benefits of personalized recommendations that we see on Amazon include:
- Increased Sales and Revenue: By providing personalized product recommendations, Amazon’s system significantly boosts sales and is generating increased sales revenue.
- Enhanced Customer Experience: Tailored suggestions create a more engaging and satisfying shopping experience, leading to higher customer satisfaction and loyalty.
- Improved Product Discovery: By implement product recommendation algorithms customers can easily find products they might not have searched for, expanding their purchasing options and increasing the likelihood of spontaneous buys.
- Higher Conversion Rates: Personalized recommendations convert browsers into buyers more effectively, improving overall conversion rates.
- Efficient Inventory Management: The system helps balance stock by promoting items that are overstocked or less popular.
- Data-Driven Insights: Amazon gains valuable insights into customer preferences and behaviors, allowing for more effective marketing strategies and inventory decisions.
Even though Amazon is an absolute giant in the ecommerce market and has a large R&D budget, smaller online stores can also benefit from the same recommendation strategy in their operations.
Implementing a custom personalization engine can revolutionize your e-commerce business by bringing the same advanced technology that powers Amazon’s success right to your platform.
Thanks to custom AI recommendation engines, even smaller stores can reap the benefits of AI-driven personalization.
These engines allow smaller retailers to offer personalized shopping experiences, boosting sales and customer satisfaction.
By leveraging advanced algorithms, small businesses can provide tailored product recommendations, enhance customer engagement, and compete more effectively in the market.
This technology democratizes access to sophisticated personalization, enabling businesses of all sizes to harness the power of AI to drive growth and loyalty.
Related Posts
- Online Shopping Recommendations – How to Introduce Them in Your Business?
- Movie Recommendation System: How It Works And How To Introduce It In Your Business
- How to Build a Recommendation System: Explained Step by Step
- Inside the Netflix Algorithm: AI’s Role in Personalizing User Experience
- Music Recommendation System: How Do Streaming Platforms Leverage AI in 2024?
Thank you for taking the time to read our blog post!