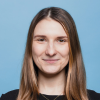
How to Boost Your Sales with a Product Recommender Tool
Looking for a product recommender to boost your ecommerce platform? This guide covers essential tools and strategies to enhance user experience, increase sales, and strengthen customer loyalty using personalized recommendations. It explores how different systems work, their immediate impact on store performance, and practical tips for smooth integration.
Contents
- Understanding Product Recommender Systems
- How to Boost Customer Satisfaction with Personalized Experiences
- How Product Recommenders Boost Ecommerce Sales
- The Use of Customer Data for Effective Recommendations
- Top Features to Look for in a Product Recommender
- Implementing a Product Recommender in Your Store
- Case Studies: Success Stories of Product Recommendations
You Will Learn That:
- Product recommendation engines, utilizing machine learning and artificial intelligence, analyze customer data to deliver highly personalized product suggestions, leading to a substantial increase in ecommerce sales and customer engagement.
- Personalization is critical in ecommerce, with proficient personalization strategies capable of increasing revenue by up to 40%. To tailor user experiences, recommendation systems analyze various data points, including user behavior, preferences, and demographics.
- Effective product recommenders boost sales by increasing average order values and encouraging repeat purchases. Key features include dynamic learning capabilities, seamless integration with ecommerce platforms, and real-time analytics for continuous performance optimization.
Understanding Product Recommender Systems
Product recommendation engines are revolutionizing ecommerce by offering customers an unparalleled level of personalization.
These powerful tools use machine learning and artificial intelligence to analyze customer data and generate personalized product suggestions.
The operation of a recommendation system involves a four-step process:
- Collection of data
- Data processing
- Filtering of data
- Generating recommendations
This process ensures the production of relevant product recommendations, tailored to the individual customer’s preferences.
The Role of Machine Learning
Recommendation engines heavily rely on machine learning for their functionality. Its algorithms are tasked with analyzing customer data to generate accurate recommendations.
As these algorithms continue to learn from user behaviors and interactions, they enhance the precision of product recommendations.
The accuracy of a machine learning recommendation system is measured by its ability to correctly predict the preferences of items for each user.
The issue of data sparsity, where users have rated only a few items, needs to be addressed to improve the reliability of recommendations by unearthing enough data to establish similarities between users or items.
Types of Recommendation Engines
Recommendation engines typically fall into three categories:
Collaborative filtering
Recommends products by analyzing user behaviors and preferences, identifying similarities between users, and inferring individual preferences from groups with shared interests or locations.
Collaborative filtering can be further divided into two types:
User-based Collaborative Filtering
The user-based collaborative filtering method finds similar users to the target user and recommends items that those similar users have liked. For instance, if Alice and Bob both liked movies X and Y, and Bob also liked movie Z, then the system might recommend movie Z to Alice.
Item-based Collaborative Filtering
Instead of finding user similarities, this method focuses on item similarities. If users A and B both liked item 1 and item 2, then the items are considered similar. Hence, if user A likes item 3, it might be recommended to user B.
Content-based filtering
Recommends products based on the characteristics and attributes of the items themselves, rather than user behavior or preferences.
This method is commonly used in movie or music platforms. For example, in a content-based movie recommendation system, the features of the movie, such as genre, director, actor, etc., can be used to describe the movie and recommend similar items.
Hybrid recommendation systems
Combine collaborative filtering and content-based filtering techniques to provide more accurate and personalized recommendations.
Content-based filtering suggests items by tracking user actions to build a customer profile and comparing it to item descriptions.
Enterprise-level businesses often adopt hybrid recommendation systems that merge techniques from both collaborative and content-based filtering to create more accurate recommendations.
Benefits of Product Recommendations for Online Retailers
Product recommendation systems offer substantial benefits, including:
- A 70% increase in purchase rates
- A 33% rise in average order values
- Product recommendations account for up to 31% of eCommerce site revenues.
- Shoppers engaging with product recommendations tend to exhibit significantly higher basket rates and per-visit spend
Source: Monetate, Barilliance
Sales and overall user experiences are improved on e-commerce platforms that utilize recommender systems to suggest items based on purchase history and viewing behavior.
How to Boost Customer Satisfaction with Personalized Experiences
For modern ecommerce, personalization is not just an added bonus; it’s a necessity
Companies that are proficient in personalization have the potential to increase their revenue by up to 40% compared to their counterparts who do not excel in this area. This shows the significant impact of personalization on financial performance.
A remarkable 71% of customers expect personalized experiences from brands, which significantly enhances their shopping experience.
Furthermore, 76% of consumers feel frustrated when they encounter a lack of personalization in their shopping experience.
Equally important, 67% of smartphone users are more inclined to make purchases from mobile sites or apps that personalize information to their location.
Machine learning integrations in recommender systems transform user data into personalized experiences, tailoring these based on individual behavior and preferences.
Sources: McKinsey, Google / Ipsos Connect
How Does the Recommendation Engine Tailor Suggestions to User Preferences
To understand individual preferences, recommender systems analyze user interaction data including:
- clicks
- purchases
- search history
- cart events
- reviews
- ratings
They leverage both implicit data, like search history and cart events, and explicit data, such as reviews and ratings, to curate suggestions. Product features, such as genre or item type, are crucial for recommendation engines to establish similarity and further personalize suggestions.
By matching the user’s previous interactions with similar item features, content-based filtering influences product visibility on product pages.
Moreover, personalization tools minimize friction, facilitating product discovery aligned with user needs.
They can boost the user experience by showing items similar to those the user has recently viewed. Displaying recently viewed products on the homepage enables returning visitors to easily pick up where they left off.
Why Relevant Recommendations are Important
The relevance of recommendations is paramount.
Hybrid recommendation models that merge content-based and collaborative filtering methods create more precise and varied recommendations, which significantly enhance user engagement.
Accurate product recommendations are instrumental in developing customer habits and modifying usage patterns, leading to improved customer engagement.
Therefore, implementing a robust recommendation engine that provides relevant and personalized product suggestions is a critical strategy for ecommerce businesses aiming to boost customer satisfaction and loyalty.
Impact on Customer Experience
The customer experience is significantly enhanced by investing in personalized recommendations.
Personalization of product recommendations aligns with individual shopping habits and customer preferences, facilitating a more intuitive and satisfying shopping experience.
Shopping carts are less likely to be abandoned when customers receive personalized recommendations that resonate with their unique tastes and needs.
Customers who encounter highly relevant recommendations show greater satisfaction, underpinned by a feeling that the online store understands their needs. Consequently, product recommender tools often lead to a more efficient shopping process by guiding the customer to items they are more likely to purchase.
How Product Recommenders Boost Ecommerce Sales
Ecommerce sales are directly impacted by product recommendations.
By increasing the average order value and encouraging repeat purchases, they significantly boost sales.
Implementing product recommendations can significantly improve the likelihood of purchases and lead to an increase in e-commerce conversion rates.
Case in point, product recommendation tools have been shown to contribute up to 35% of Amazon’s sales, demonstrating their powerful influence on revenue growth.
Incorporating personalized recommendations into marketing strategies not only increases customer satisfaction but also directly correlates with an uptick in retention rates and sales.
Increasing Average Order Value
Increasing the average order value is one of the key benefits of recommendation engines.
They facilitate upselling and cross-selling by showing customers high-end products or related accessories.
By providing personalized recommendations, users can easily discover products that match their preferemces, trends, interests, and behavior. This in turn aims to increase upsells, cross-sales, cart sizes, and average order values..
In fact, personalized product recommendations have been reported to increase average order value (AOV) by 10%, according to Salesforce. Companies that have implemented recommender systems typically see an increase in upselling revenue by 10-50%.
Encouraging Repeat Purchases
Improvement in customer loyalty, leading to repeat business and increased customer retention, is an important benefit of personalized recommendations.
Customized experiences make customers feel valued and appreciated, encouraging loyalty and repeat business.
Product recommendation engines enhance customer retention by making users more likely to stay loyal through personalized suggestions.
Amazon’s recommendation engine is a prime example of how understanding and predicting user interests and behaviors can drive purchases, increase cart volume, and reduce cart abandonment.
The Use of Customer Data for Effective Recommendations
Effective recommendations rely on leveraging diverse customer data.
Access to user data, such as:
- buyer personas
- purchase habits
- traffic source
- the device used for interaction
is pivotal for powering smarter recommendations.
By analyzing behavior data collected from online stores using JavaScript APIs, recommender systems are able to provide dynamic product recommendations. Demographic data such as age and gender, combined with psychographic data regarding interests and values, augment the accurate segmentation of similar customers for improved recommendation accuracy.
Purchase History and Online Behavioral Data
Personalized product suggestions are created by recommendation engines by integrating past purchases and browsing habits.
They analyze a user’s browsing habits to curate product suggestions that resonate with their individual online behavior and interests. Platforms like Adoric use real-time data, considering factors such as:
- how a user interacts with the website
- the user’s demographics
- the user’s location
- the user’s purchase history
This information is used to create suggestions that are current and relevant to each individual user, taking into account the preferences and behavior of similar users and other users.
Customer feedback and reviews are vital inputs for recommendation engines, aiding retailers in enhancing future recommendations and building stronger customer relationships. Algorithms use data like location, gender, and purchase intent to display relevant product recommendations, adding a layer of personalization to the shopping experience.
Segmenting Customers for Targeted Marketing
Targeted marketing campaigns, which are more likely to resonate and engage customers, can be achieved by segmenting customers based on interests and behavior.
Customer attribute data such as: age, gender, interests, values are critical for segmenting customers effectively.
Segmenting customers enables the delivery of more personalized product suggestions that align with the specific user’s characteristics and preferences. The more targeted the marketing campaign, the more effectively it engages customers and leads to conversions.
[Read also: Netflix Algorithm: How Netflix Uses AI to Improve Personalization]
Top Features to Look for in a Product Recommender
There are some key features to consider when choosing a product recommender.
A versatile product recommender should feature a range of algorithms such as ‘frequently bought together’, ‘viewed together’, similar, bestsellers, trending, and cater to different product discovery needs like hot products or best cross-sell relevant products.
Advanced recommendation engines, like these provided by Stratoflow, use machine learning technology for high-performance recommendations and adjust strategies in real-time in response to customer journey changes, enhancing the adaptability of product suggestions.
Deep attribute matching allows new products to immediately benefit from existing data patterns, streamlining product testing and shortening the time to effective recommendation.
Additionally, it is crucial to look for a tool that does not suffer from the cold start problem but can display recommendations immediately. This capability ensures that even new users or products with little to no interaction data are provided with relevant suggestions right from the start.
Searching for a custom recommendation engine? Schedule a call with our machine learning experts today!
How to Integrate Product Recommender System with Ecommerce Platforms
When selecting a product recommendation tool, it’s critical to ensure easy installation and seamless integration with the ecommerce platform.
For example, you can choose from out-of-the-box recommender systems from marketplaces like Shopify. This enables you to leverage powerful recommendation tools without the need for complex coding or integration processes.
However, for greater personalization and customization tailored specifically to your store, it is worth considering a recommendation engine built by specialized companies, such as Stratoflow. These specialized solutions can offer more advanced features, deeper integration, and higher adaptability to your unique business needs, providing a more refined and effective recommendation experience for your customers.
[Learn how to build a recommendation system here]
Real-Time Analytics and Merchandising Control
For the effective management of a product recommendation engine, the following are crucial:
- Real-time analytics: Dashboards that offer real-time analytics that include key metrics such as responses, payments, completion rates, email open rates, and response summaries.
- Merchandising control: This allows you to have control over the products that are recommended to users.
- Algorithms: Algorithms are fundamental to recommendation systems as they determine the speed at which profits are generated from product suggestions.
Continuous monitoring and adjustments to a product recommender system are necessary, focusing on metrics aligned with business goals to maintain effective performance.
Implementing a Product Recommender in Your Store
Several key steps are involved in implementing a product recommender in your online store. The process typically involves:
- Platform configuration
- Integrating codes provided by the engineering team
- Adding and setting up data
- Creating product recommendation banners/widgets
- Selecting the right algorithm for generating product recommendations.
Some providers like Alie offer a simpler alternative for recommendation engine integration, with a five-step process that requires no additional coding and includes support at each stage, offering a streamlined integration method for any website.
Customization and Testing
For a successful product recommendation strategy, customization and testing are key components.
Customization of a product recommendation engine involves configuring it to match the website’s specific needs and data fields, including the selection of various algorithms to tailor recommendations.
Tools such as Google Optimize, VWO, and Optimizely offer A/B testing capabilities, allowing businesses to test and optimize various aspects of their product recommendation engines for improved performance.
Case Studies: Success Stories of Product Recommendations
At Stratoflow, we’ve seen a remarkable business opportunity in intelligent product recommendations for the e-commerce industry.
That’s why we decided to build Recostream – an advanced AI-powered custom recommendation engine for online stores of all sizes.
Recostream’s mission was to provide advanced, data-driven recommendation models that could be easily integrated into any e-commerce platform or mobile application without requiring technical resources from customers.
Their solution was to augment AI/ML-driven models with easy integration methods, allowing non-technical users to benefit from advanced analytics and A/B testing capabilities.
The results were remarkable: most e-commerce stores saw a 5-10% increase in sales with Recostream’s recommendations.
This success story underscores the importance of working with professionals experienced in developing such systems, as they can provide solutions that are not only innovative, but also easy to use and effective in driving business growth.
For companies looking to gain a competitive edge and improve the customer experience through tailored recommendations, investing in a custom recommendation engine like Recostream can be a game changer. Now Recostream is part of a GetResponse product suite.
The expertise and experience of professional developers with sufficient programming and data science skills can transform your company’s approach to customer engagement and sales strategies.
If you’re considering developing a custom recommendation engine, get in touch with us!
Summary
In conclusion, product recommender systems offer a powerful way to personalize the shopping experience for customers, leading to increased sales and customer satisfaction. Through the use of machine learning and AI, these systems analyze customer data to generate accurate, personalized product suggestions. By increasing average order value and encouraging repeat purchases, they boost ecommerce sales significantly. Implementing a product recommender involves several key steps, including platform configuration and data setup. It’s important to choose the right tool that aligns with your specific business needs and to continually monitor and adjust the system for optimal performance. With successful implementations by companies like Best Buy and ASOS, it’s clear that product recommender systems are a worthwhile investment for any ecommerce business.
Frequently Asked Questions
What is a product recommender system?
A product recommender system uses machine learning and AI to analyze customer data and provide personalized product suggestions.
How do product recommender systems work?
Product recommender systems work by analyzing customer data, including past purchases and browsing habits, to understand individual preferences, and then use this information to recommend products aligned with those preferences.
What are the benefits of product recommender systems for online retailers?
Product recommender systems can help online retailers increase purchase rates, average order values, and overall revenue growth, while enhancing the customer experience through personalized suggestions.
What key features should I look for in a product recommender system?
Look for dynamic learning capabilities, seamless integration with ecommerce platforms, and real-time analytics in a product recommender system to make an informed choice.
How can I implement a product recommender system in my online store?
To implement a product recommender system in your online store, you’ll need to configure the platform, set up the data, and customize it to fit your website’s requirements.
Related Posts
- Types of Recommendation System For Business Growth: Your Guide
- An In-Depth Guide to Machine Learning Recommendation Engines
- How to Build a Recommendation System: Explained Step by Step
- Key Benefits of Artificial Intelligence For Modern Businesses
- Future of Business Intelligence: Emerging Trends in 2024
Thank you for taking the time to read our blog post!